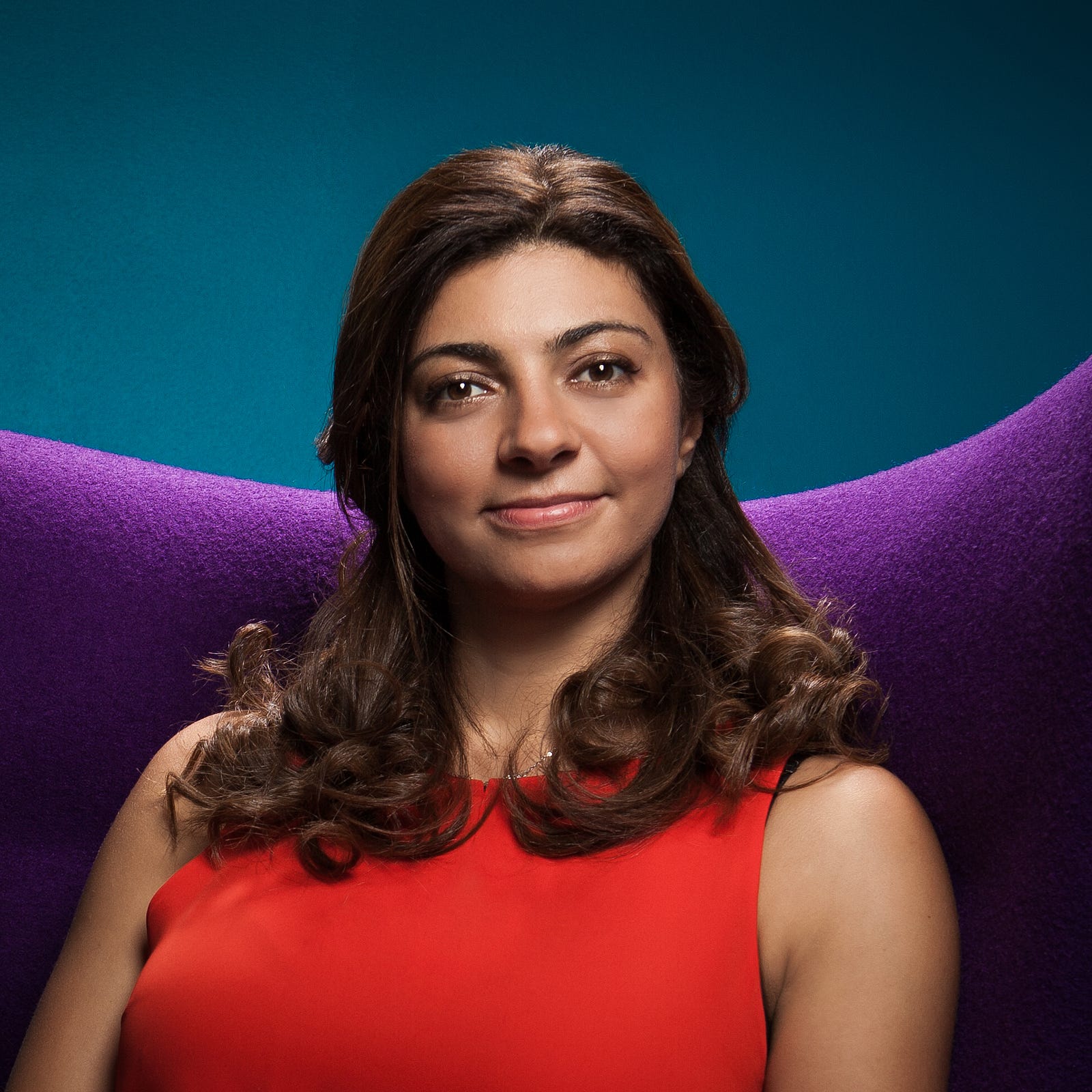
5 Questions with Affectiva’s Rana el Kaliouby
In the quest to digitize every aspect of a business and convert every action into data, we often overlook human emotion. But emotion is key to everything from sales and marketing to human resources. As the old maxim has it, we buy based on emotion and then rationalize it later. Is there some way to convert emotion into data too?
That, in a nutshell is Affectiva’s raison d’être. The Waltham, Mass., company uses computer vision and deep learning to analyze consumers’ facial expressions and translate that into raw data. There are multiple ways to use such technology. Fight The Stroke employs it to help stroke victims learn or relearn motor skills. Giphy uses it to tag GIFs by their emotional content. Other uses touch on entertainment, education and advertising.
The woman behind Affectiva is Rana el Kaliouby, an Egyptian-born computer scientist. As she explained in a 2015 TED talk, when she was a student at Cambridge University, el Kaliouby noticed that it was difficult to convey emotion over Internet-based communications. (Emoticons didn’t cut it.)
That led to further work as a research scientist at MIT. El Kaliouby continued to study emotion and created Affectiva, which was spun off from the MIT Media Lab in 2009. El Kaliouby recently took time to answer Deeply AI’s Five Questions. Here’s an edited version of that conversation:
What is the biggest challenge facing the AI industry right now?
One of the biggest challenges facing the AI industry is data collection. To date, Affectiva has analyzed 6 million faces in 87 countries, but we are constantly training our algorithms, requiring more and more data. And it’s not just about the amount of data, it’s about the quality as well. Real-world data collected outside of the lab is the most ideal, because it will reflect the real-world conditions people will be under when interacting with AI-integrated systems. The problem is, data collection at this scale is difficult to come by, especially if it is challenging to collect.
Look at the automotive industry for example. With AI-enabled cameras pointed toward a driver’s face, automakers can determine if a driver is tired or distracted, and take steps to make them alert, potentially saving the driver’s life and many other lives. This data is best collected out on the roads where drivers are interacting with other real-world drivers, not in a controlled setting. So for AI startups, tech giants or leading automakers alike, gaining access to this kind of data is difficult. The company that is able to solve this challenge will gain an advantage over the competition, in the world of AI and other industries like automotive as well.
Where do you see AI in five years?
Over the next five years, AI will enable increasingly personalized and relational interactions between people and technology. For consumers, this means more engaging digital experiences, and for brands, AI can be a big differentiator when it comes to the customer experience.
Take the automotive industry. What differentiates one auto brand from the next? A lot of it has to do with the customer experience in the car. With AI, rather than being just a mode of transportation, cars are becoming conversational interfaces between the driver, passengers and vehicle itself. Cars will monitor passengers’ cognitive state for two things, the first being driver safety, which includes their level of distractedness, drowsiness, road rage, etc. The second is for personalization of the in-cabin experience, which means analyzing thoughts and feelings, so that the car can tailor things like infotainment, route recommendations, temperature control and more, according to the passenger’s preferences.
It doesn’t stop there. The auto industry’s focus on AI is particularly pertinent as semi-autonomous and fully autonomous vehicles come to the fore. Specifically, AI’s role in solving the “hand-off” challenge. An autonomous’ vehicles AI will have to decide when a “hand-off” should take place to pass control between the vehicle and a human driver. For Level 3 and Level 4 vehicles, this decision will be a matter of life or death. The decision will be made using computer vision and multi-modal AI that is measuring a person’s facial expressions, voice, gestures, body language and more, to ensure the driver is awake, alert and engaged.
With AI, rather than being just a mode of transportation, cars are becoming conversational interfaces between the driver, passengers and vehicle itself.
What’s the most interesting AI startup you’ve seen?
I think the work we are doing here at Affectiva with Emotion AI is an important piece of the AI puzzle that the industry is trying to solve, as AI increasingly comes to the fore, and we expect these systems to perform increasingly complex functions in our daily lives. I started Affectiva with the vision of creating emotionally responsive machines, which led to the development of what we now recognize as artificial emotional intelligence — or “Emotion AI” for short. This technology has been applied across verticals such as automotive, robotics, conversational agents, healthcare, market research and more, and it’s been fascinating to see the range of experiences that can benefit from not just AI with a high IQ, but EQ as well.
I also recently met Jean-François Gagné, the co-founder and CEO of Element AI and was very impressed with their vision of bringing AI to enterprises.
How do you define AI?
Artificial intelligence is a field of study concerned with giving machines intelligence, of which there are many flavors: computational intelligence, cognitive intelligence, conversational intelligence, social intelligence, emotional intelligence and more. There’s also artificial general intelligence, which refers to having a machine that can successfully perform any intellectual task that a human being can.
The way you build artificial intelligence is through machine learning. Machine learning approaches allow systems and algorithms to automatically learn and improve from experience — massive and realistic data — without being explicitly programmed.
Thus AI systems, narrow or generalized, are powered by data and machine learning methods, such as deep learning or reinforcement learning. In a world of AI hype, where many companies claim to be AI, understanding the underlying approach and their use of machine learning serves as a great litmus test.
What books, blogs, etc., do you read to stay on top of what’s going on in AI?
Well — I breathe AI day in, day out. Not only at Affectiva, but through other activities I am involved in. For instance, I am a World Economic Forum Young Global Leader and in that capacity I serve on the future global council on robotics and AI. I am also a member of the Partnership on AI, the AI consortium started by Microsoft, Google Deepmind, Amazon, Apple, Facebook and others to ensure that AI is applied in a way that benefits society. So I find that I am in the know when it comes to advancements in AI (I am often more concerned about what is happening in other areas, where I am not as immersed, like blockchain!)
Having said that, there are a number of people I admire / follow closely in the AI space. Top of mind are: Andrew Ng; Eric Horvitz, head of Microsoft Research; Fei Fei Li at Stanford/Google; Demis Hassibis of Google Deepmind. I also follow CBInsights for news around investments in AI and top AI startups. In terms of companies, I’ve been following what NVIDIA has been doing in AI and automotive for a while now, and I’m excited that Affectiva is now working on an integration with them.
There are also some events that are focused on what’s happening in the AI space: the O’Reilly AI conference is one of my favorites. Ben Lorica and his team have a great vision for that conference and its a good mix of research and applied AI. I’ve been to and spoken at all of them so far.